Situation
Our client, a US-based multinational food conglomerate, wanted to improve the accuracy of its trial, repeat, and yearly sales predictions.
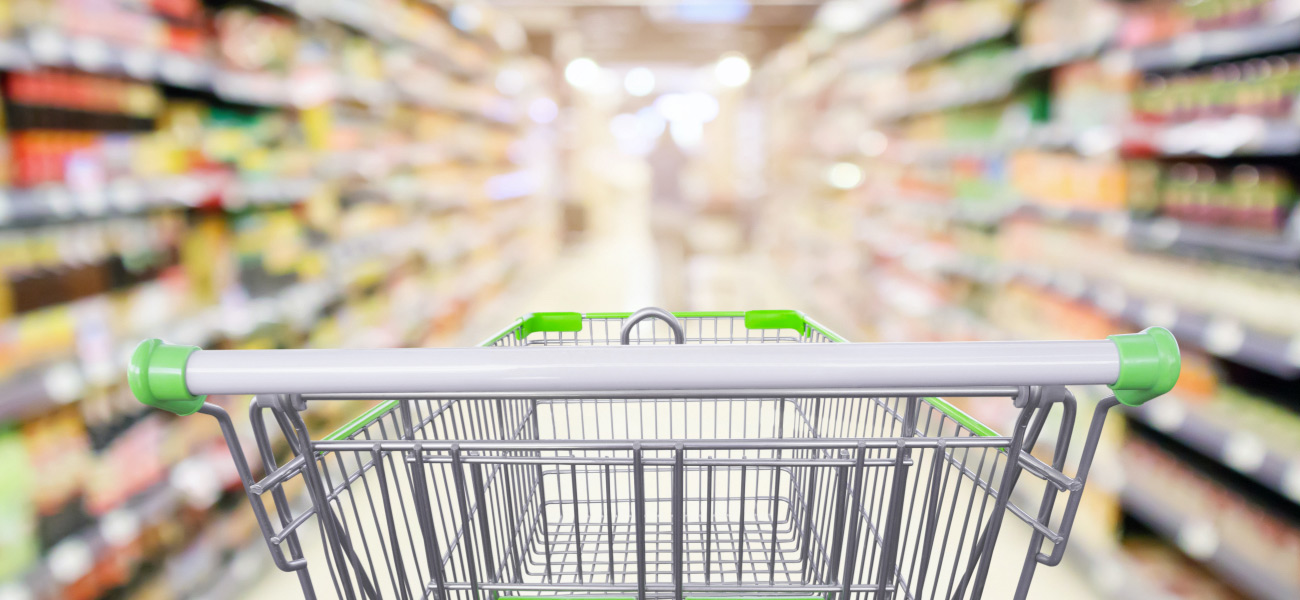
This multinational food firm needed to harness millions of data points to predict consumer reaction to new products.
The company needed a way to harness millions of data points and use sophisticated predictive modeling techniques to predict how consumers would likely react to a new product, which would help it mitigate the risk of launching new products.
Action
We created a digital twin, a highly complex model of a physical entity—in this case, our client’s consumer base. Its detailed nature allowed experts to forecast changes and reactions accurately.
Data came from multiple sources, including customer raves and complaints, disaggregated panel data, trial/repeat data, in-market sales, media spend, distribution, and more.
We developed advanced parametric time series models for trial, repeat, and additional repeat purchases over a monthly period for one year. Then we used a clustering algorithm to find groups of new products that had different drivers but similar market performance projections. We used these clusters to evaluate how the success of new products would impact the cluster as a whole. We then built an AI- and ML-based regression model to predict sales and market share for individual products.
Results
Now our client can isolate multiple factors and drivers of customers’ acceptance/rejection of a product, which lets it create dynamic benchmarks for new products and simulate product performance based on different targets, need states, and product characteristics.
Our client can also tweak offerings and gauge consumer reaction before launch, which has the potential to save huge amounts of time and money.
Advanced modeling and the ability to use multiple data sources enable our client to achieve greater accuracy in its new product predictions.
Simulating product performance combinations and their impact is much easier, as is identifying which variables affect products’ success.
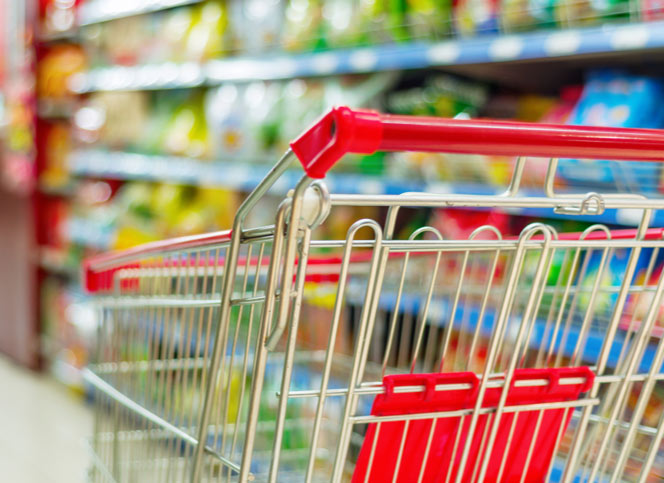
We used panel data, trial/repeat data, in-market sales, media spend, and other data.
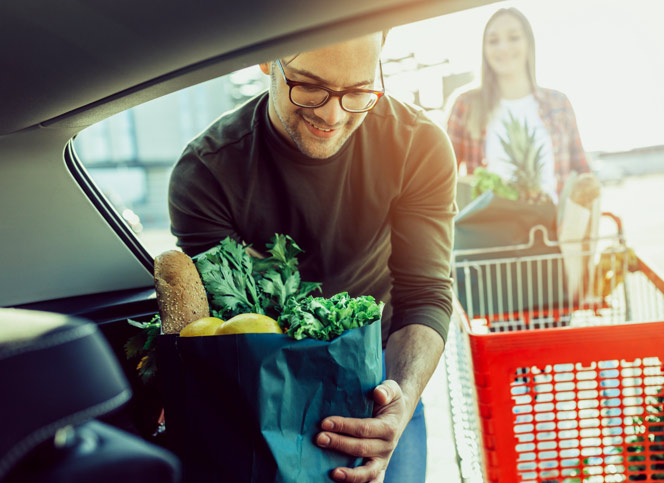
Our regression model used AI and ML to predict sales for individual products.
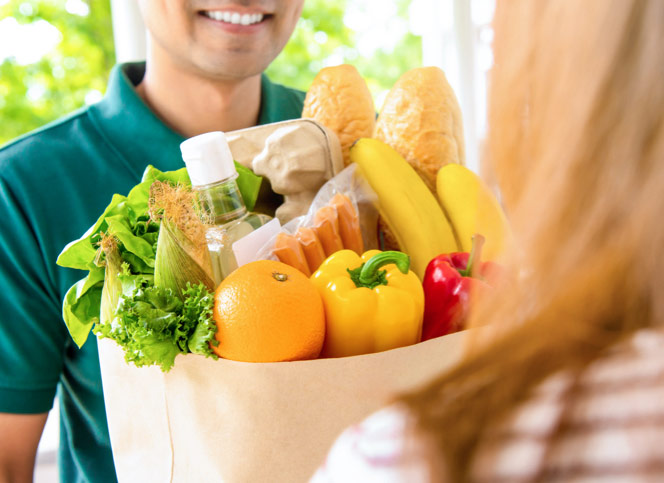
Our client can isolate multiple factors and drivers of customer response to products.
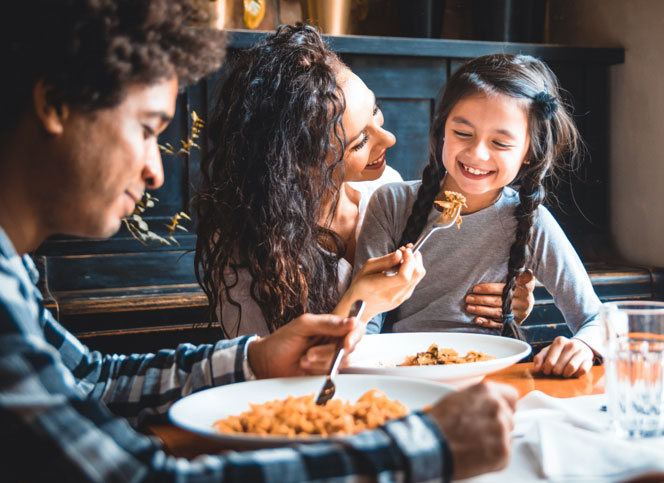
Our client can tweak offerings and gauge consumer reaction before launch.
-
90%
accuracy predicting sales -
-
1-year
trial period -
-
1
dashboard isolates multiple factors
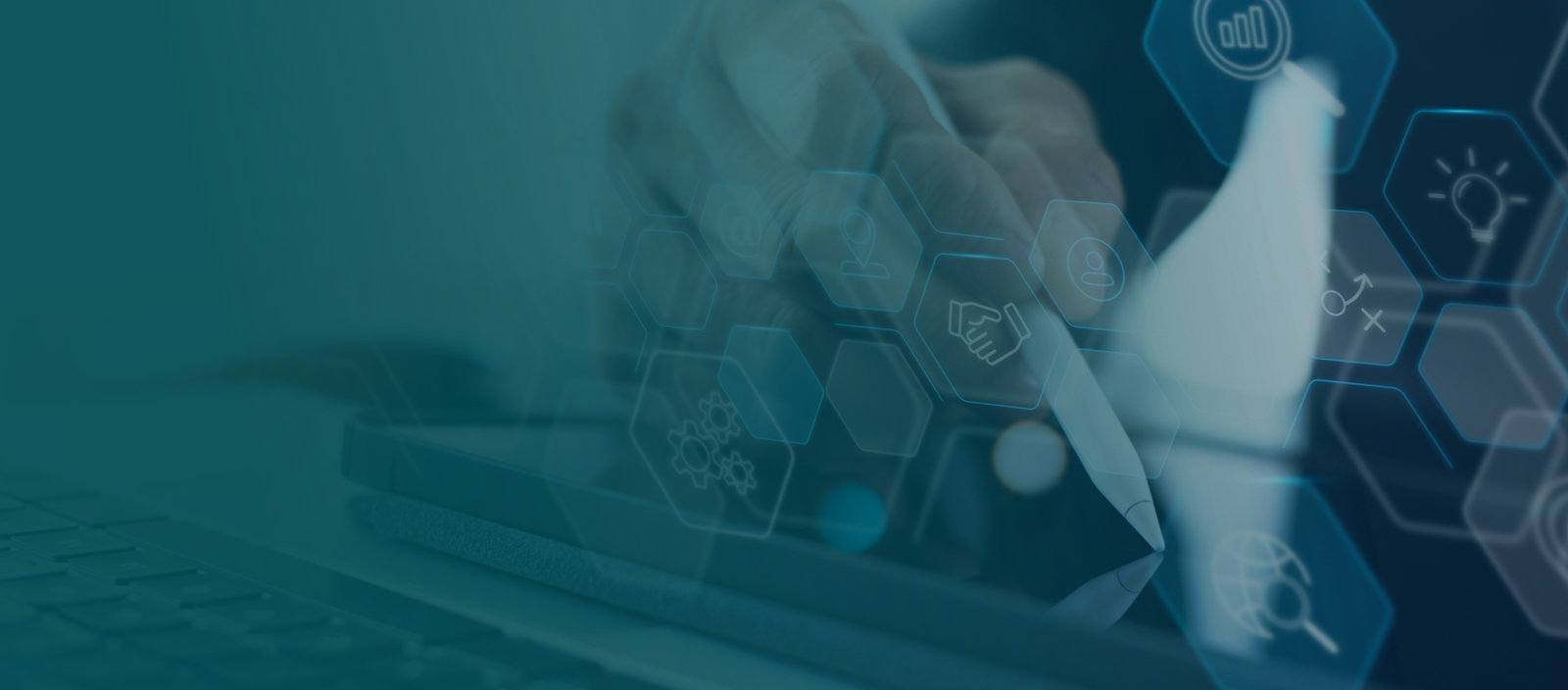
Drives results across industries
NAVIK AI solutions for Sales, Marketing, Operations, and Technology turn data from across your organization into insights that drive revenue, efficiency, and results.
Deepen your insights