- Posted on : October 16, 2024
-
- Industry : Corporate
- Service : Corporate
- Type: Blog
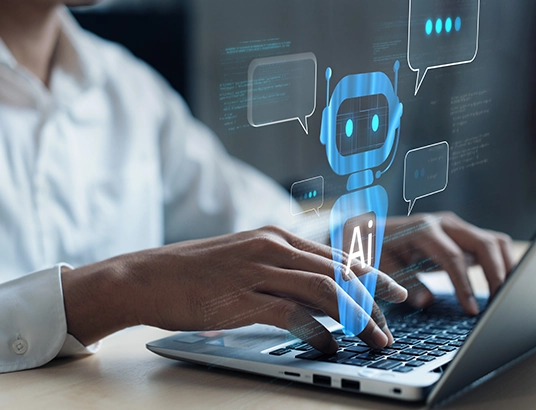
Crafting the perfect prompt for AI is akin to composing a symphony. Each word and detail must resonate in precisely the right way to evoke the desired response. Precise, actionable prompts transform your AI interactions and make them more insightful, relevant, and effective, which maximizes their value across your company. Vague or unclear prompts waste scarce time and resources. They don’t provide usable results and leave you to complete the original task manually.
The best approach to prompts is to give the AI the clarity and insight into the task that you would want if you were assigned the task yourself. Precision, data, clarity, and successful case studies are enablers, whereas ambiguity and vagueness pose unwarranted hurdles. Once you adopt this human-centric approach to writing AI prompts, the quality of the product that your AI-enabled solutions provide will radically increase.
Here are eight ways that we’ve learned to apply our human-centric approach to writing AI prompts:
Define the speaker and the audience.
The world’s top ad agencies and marketing organizations live by this. The speaker who demonstrates knowledge of the reader and their situation instantly has more credibility than the speaker who doesn’t. This creates the connection that drives business results.
Example: “You are a veteran business consultant who specializes in helping small-company CEOs who are reaching retirement age attract buyers who will value their life’s work and continue their traditions.
Please draft an email suitable for CEOs who run low-tech manufacturing companies in the American Rust Belt and who make paper products, building materials, and specialized industrial equipment. Outline three direct mail strategies you can help them execute to sell their businesses and structure the proceeds to support an extended family business.
See the attached email threads and strategy decks for details.”
Divide and conquer. The strategy of breaking one big problem into many small ones has worked for centuries. In the 21st century, it keeps the AI from getting overwhelmed. It also lets you see results arrive incrementally so you can identify errors and hallucinations before they propagate into far bigger problems elsewhere.
Bad Example: “Write a 2,000-word article on avoiding ultra-processed foods (UPF).”
Good Example: “Write a 2,000-word article entitled “How to eliminate ultra-processed foods from your diet.” The structure should be
- Executive summary of the entire article. 500 words in length.”
- Define UPF per the Nova classification system. 500 words.
- Define the processes and additives that turn foods into UPF. 500 words.
- Describe the reasons that businesses create UPF. 500 words.
- Talk about the health benefits of avoiding UPF. 500 words.
Be specific.
Tell the AI exactly what you want: format, output length, level of detail required, style, tone, etc. Also provide detailed context for your request.
Example: “Please write an outline for a report that identifies and analyzes the top five post-pandemic trends in B2B and B2B2C marketing for manufacturing companies serving the transportation sector. Write this outline to follow the standard format: executive summary, introduction, as many sections as needed to address the top five trends and how they’re related to one another, a conclusion that summarizes the report, and an appendix of citations.”
Show it what you like.
Giving the AI an example of what you want reduces ambiguity, essentially giving it a head start to provide the exact response you need. You could include inputs from branding and style guides, tone and voice guidance from marketing teams, pieces of code, document and other templates, or pieces of content that are close to the result you want AI to provide.
Example: “Create a comparison table for two ruggedized tablets. The table should make sense to people who are not technologists. Categories: Price, Key Features, User Reviews, and Support Plans. Under Key Features, list display size, storage, drive type, software, case material, and processor size.”
Specify the form factor.
Tell the AI the exact format that it should use to structure its response. That could be a talk track for a PowerPoint presentation, a series of bullets for executives to use as talking points, a sales enablement deck, or a text summary. The sky is the limit on this. Including examples as described above is a great shortcut for this.
Example: “Please provide a series of talking points from the attached decks that our CMO can use to address a group of Ph. D. students who are sequencing the human genome looking for mutations that cause cancer. Focus on how our technology, especially our storage solutions, is ideal for applications that require vast amounts of data. Cite our work supporting CGI animation for superhero movies that resonate with this audience.”
Don’t be negative.
Telling an AI-enabled solution what to do is more productive than telling it what to avoid. Just like humans, AI must invert negative logic to process it. This introduces the potential for errors in understanding your intent. Positive instructions don’t need to be inverted, leading to better outcomes. The more clearly you state what you want, the more likely the AI is to provide it.
Examples:
“Only use climate data gathered after 1887” is better than “Avoid climate data from the Little Ice Age.”
“Write in language suitable for audiences with a high-school education” is better than “Avoid using industry-specific terms.”
“Be concise” is better than “Omit details that won’t matter to this audience.”
Provide data—lots of data.
Just like people, AI needs insight into the context of your request to provide useful answers. This is even more important when you need AI to help you understand trends and patterns.
Precise, relevant data like dates, categories, prices, values, weights, and other specific figures is critical, and the more specific you can be, the better the answers you’ll get.
Example: “Please analyze failure rates of Products A, B, and C over the last six months as detailed in the attached PowerPoint deck and PDFs. Please identify the leading causes of failure, the five most common conditions under which each product is most likely to fail, and the most common remedies that our teams have implemented in the field. Please provide a three-paragraph summary of these issues using language that non-SME audiences can understand.”
Make the AI tell you what it’s thinking.
Asking for detailed reasoning that supports a response is called chain of thought prompting. When done properly, it helps you judge how well the answer is suited to your needs.
Example: “You’re a copywriter and content strategist who must revise a technical report for members of a 14-member purchase committee at CORPORATION X. All committee members are experienced businesspeople, but only 25% are technologists per se. The others are in roles focused on growth, innovation, commerce, and operations.
[report]
Use the following criteria to explain your response:
- Relevance: Describe how much of the data in this report will be relevant to the Chief HR Officer and Chief Legal Officer of CORPORATION X and why. Focus on definitions of "personal data", "processing", "data subject", "controller", and "processor" as stated in Article 4 and other relevant portions of the GDPR.
- Architecture: Describe how and why you simplified the solution architecture diagrams.
- Simplification: Discuss how you restated the technical concepts presented here to make them more easily understood. Focus on the shift to data lakes from their current storage solutions.
- Promotion: Detail an integrated campaign for CHROs and CLOs that sharing this document via blogs and other updates to our current website, LinkedIn and other social media, video, and email.
- Describe a roadmap for keeping this document up to date, and outline a process for modifying it to be relevant to the CHRO and CLO of CORPORATIONS Y and Z.”
Conclusion
Know the limitations of AI.
It's still widely misunderstood. Many people, especially those outside of tech orgs, expect wonderful things from a magic black box. Some of what AI can do borders on the magical, but there are limits.
For one thing, it’s unable to process data in real time, since its knowledge is only as current as the last training cut-off.
It can’t access or retrieve personal data that wasn’t shared during the interaction, nor can it interact directly with external software, databases, or live web content.
There is a chance that your AI can learn bias from training models then inadvertently replicate it in work product. It also has a limited understanding of context, and it can’t provide deeply nuanced responses to complex or ambiguous prompts. Perhaps its biggest failing is its inability to form genuine, empathetic connections with real humans.
As a user of AI, you’ll soon realize and be able to work around these limitations, but stakeholders in the business may not. It will be your job to help them navigate the hype and understand the limitations of AI as it exists now.
Learn by experimenting.
Prompt engineering remains an emerging field. As you work within it, learn to see each prompt as a hypothesis, then test it by examining the results it provides. Focus on how the quality and significance of each prompt changes the work, and whether the results provided match the magnitude of the change you expected.
Again, the quality of the prompts matters most. In theory, the words “Have a nice day” and “Enjoy the next 24 hours” mean the same thing. But to readers, the former is a pleasantry while the latter is a threat. Less can easily be more, and more can easily be less.