- Posted on : February 7, 2024
-
- Industry : Corporate
- Studio : Data & AI
- Type: Blog
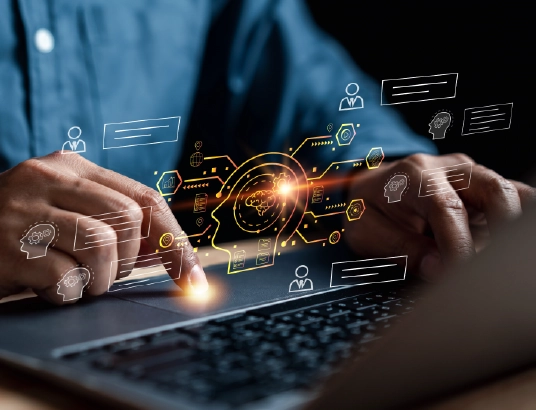
A whirlwind year in AI
In 2023, we witnessed the meteoric rise of generative AI. ChatGPT dominated the headlines and, in the process, became one of the fastest growing consumer applications of all time. In a pattern witnessed throughout my career in business technology, trends that start in the consumer world quickly trickle into the business side. With 2023 safely in the rear-view mirror, I'd like to share some lessons I learned in the enterprise AI space from the year gone by and speculate a bit about what we will see in 2024.
What we learned along the way
At Infogain, we had already developed a machine-learning powered business solution called ASK NAVIK. The data science engine behind that solution was fuelled by an "old school" Natural Language Processing (NLP) algorithm. We quickly recognized that large language models (LLMs) would wholly disrupt the enterprise chatbot market and re-built ASK NAVIK with an LLM.
So, as the product leader for an enterprise AI platform, what did I learn from the rollercoaster ride that was 2023? I will boil down our learnings to three significant areas:
- Customizing LLM generations is critical for business use cases
- LLMs aren't built for structured data… yet
- Total cost of ownership is difficult to predict
1. Customizing LLM generations
It isn't all that difficult today for an enterprise to get started with generative AI. Powerful foundation models are available today from all three hyperscalers, and for the more ambitious, open-source models with billions of parameters can be easily acquired (and less easily fine-tuned) for business use.
What is more difficult is ensuring that these models produce generations that are:
- Relevant
- Speak the language of your business
- Adhere to the business rules and regulatory requirements
A relatively straightforward approach to enriching the capabilities of an LLM with your organization's data is using retrieval augmented generation (RAG). This enhances what the LLM can produce on its own by augmenting its powers with your data. And now, various approaches exist to do this in a highly secure way where your data never leaves your firewall.
What is more challenging is to build both input and output layers that ensure the LLM produces appropriate content. For instance, responses should be consistent, adhere to certain business rules, and comply with use case boundaries. In our ASK NAVIK platform, we are investing heavily in both a question analyzer module that ensures prompts are routed optimally and a response generator layer that ensures generations speak the language of our clients' business.
2. LLMs aren't built for structured data… yet
Today's LLMs are fantastic at working with unstructured text data but struggle to conduct basic calculations with structured numerical data. Structured data use cases get even more complicated when we need to comply with various business rules.
A simple example of this is what we encountered while building a PoC of ASK NAVIK for Infogain's finance organization. Our colleagues required generations to be produced with very particular numerical formatting and required reports across multiple currencies. There are many other examples here, i.e., ensuring the generations stay within the boundaries you have defined for the use case, mandating generations conform to particular branding requirements, and guaranteeing that a generation complies with corporate ethical standards.
Gen AI business use cases require an input/output orchestration layer.
Here is another example of why input and output orchestration is critical to succeed with enterprise generative AI use cases. On the input side, prompts must be analyzed to ensure they are routed appropriately through the system. For instance, prompts requiring structured data may need to be translated into a SQL call. On the output side, we can define and enforce various business rules and now reassemble the generation to ensure its accuracy and relevance. For instance, we may need to combine structured data from a database with text-based content being pulled from a knowledge base. Check out this demo recording to see the capability in action.
3. Avoiding TCO surprises
At first glance, the cost of designing, developing, and managing a generative AI solution seems reasonably clear. Most organizations have a couple of decades worth of experience building web-based applications and can reasonably estimate the cost of a new application. Where things get trickier for generative AI solutions is the cost of token consumption.
Generative AI systems operate on a token-based framework, where costs are linked to the number of tokens processed during language generation. The model may initially appear similar to other usage-based software pricing, but the complexity lies in the dynamic and context-dependent nature of token usage. Tokens associated with any particular generation will be influenced by factors such as the length and complexity of user inputs, varied language structures, and the diversity of generated content.
We have taken a couple of different approaches with ASK NAVIK to drive down costs and improve the transparency of consumption. The first point is to recognize that different LLMs can have dramatically different cost structures. For example, estimates indicate that the cost of GPT-4 is approximately 22X more expensive than the ChatGPT API (powered by GPT-3.5-turbo).
Coming back to the ASK NAVIK input layer, we can divert prompts requiring less sophistication to the GPT-3.5-turbo-powered API and leverage GPT-4 only when needed. The second approach we have developed is to show real-time token consumption within the ASK NAVIK interface. This can help the user understand the cost of usage on a relative basis. Providing awareness of consumption along with educating users can contribute to cost reduction.
What do we have in store for enterprise AI in 2024?
From the side stage to the spotlight
I anticipate that the central theme in enterprise AI this year is a shift from experimentation to operationalization of business AI solutions. We are seeing early promise for use cases that enhance employee experiences, drive sales and marketing productivity, and deliver superior customer experiences. Ambitious businesses are already partnering with AI service providers or working on solution prototypes themselves.
In 2024, these experiments will move out of the lab, and I anticipate two themes will emerge:
- We will see organizational-wide deployments of the most promising use cases.
- These deployments will further extend into adjacent business processes and IT systems.
I will provide a specific example of how this evolution will play out at Infogain. We will soon launch ASK NAVIK in our employee HR portal. The HR deployment will provide a chatbot-like experience for employees to search and engage with HR policy documentation. Phase One of this deployment will include essential integration with our HRIS systems to ensure ASK NAVIK produces content relevant to the employee's geographical location. Phase Two will consist of much deeper HRIS integration to provide highly personalized insights related to employee leave time remaining, training hours consumed/required, promotional paths, salary information, and much more.
Future-proofing is impossible. But...
History is not always predictive of the future, but I am confident one theme from 2023 will continue into 2024. The market and the technology powering it will continue to move at lightspeed. As a product leader in the AI space, my main takeaway is to focus on where we can add value for our clients.
For instance, it is likely that the technology we use today to power enterprise retrieval augmented generations (RAG) will soon be obsolete. We are already seeing this with OpenAI's release of the Assistants API. This API automates some of the technology that many organizations – including Infogain – use to power RAG use cases, such as a chunking algorithm and creating embeddings to be stored in a vector database.
But today, most organizations still need help with RAG-based use cases, and there may be a wide variety of reasons (cost, privacy, trust, control…) they have not yet adopted a solution like the Assistants API.
In conclusion
For those of us building in the enterprise AI space, it is impossible to future-proof our products. I suggest staying grounded in your clients' needs, listening carefully to their wants, needs, and aspirations, and meeting them where they are ready to engage.